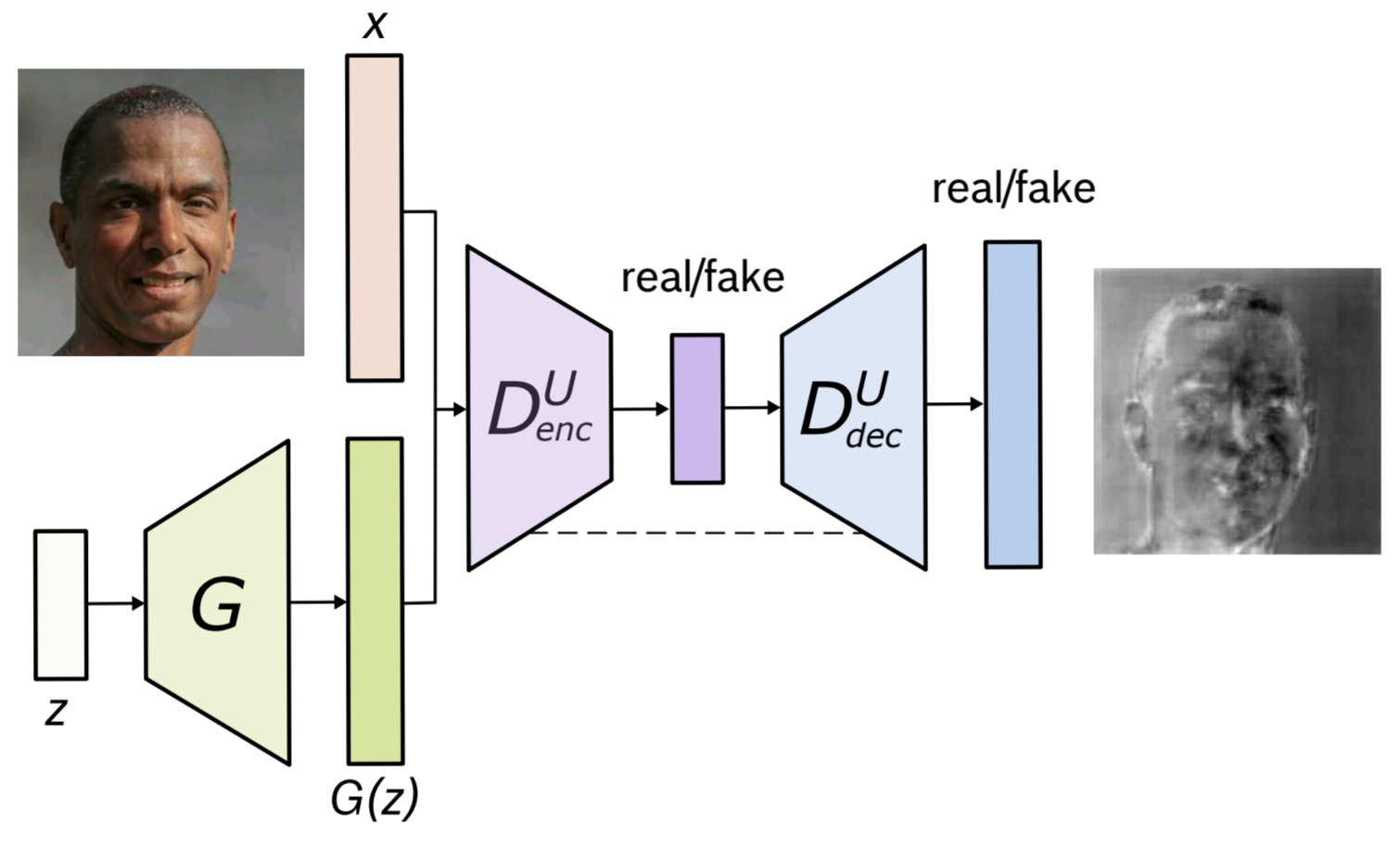
This article will discuss ML, clustering and metadata. It will also explain the difference between supervised and unsupervised learning, as well as what metadata is. We'll also cover how to create a Metadata registry that stores your ML model's metadata. These concepts are key to understanding ML models. They can be used to help you build better models. This article will cover these concepts in greater detail.
ML model metadata
Metadata is a critical component of ML models. This allows for auditing and reproducibility. A metadata management tool lets you store and retrieve all the data and settings for your model in one location. Metadata can help you identify reused model building steps, assimilate models, and allow for auditing and comparison. ML model metadata includes information such as model type, types of features, preprocessing steps, hyperparameters, metrics, and training/test/validation processes. It also includes details such as training time and number of iterations.
These data are often kept in a repository and can be linked to the model through one or more edge computing devices. You can connect a camera or microphone to the ML version 400 with Bluetooth communications. The raw input data can be stored at the ML model repository 488, where it is associated with labeled labels or expert input. These data may also be stored in another location that is accessible to the ML engine.
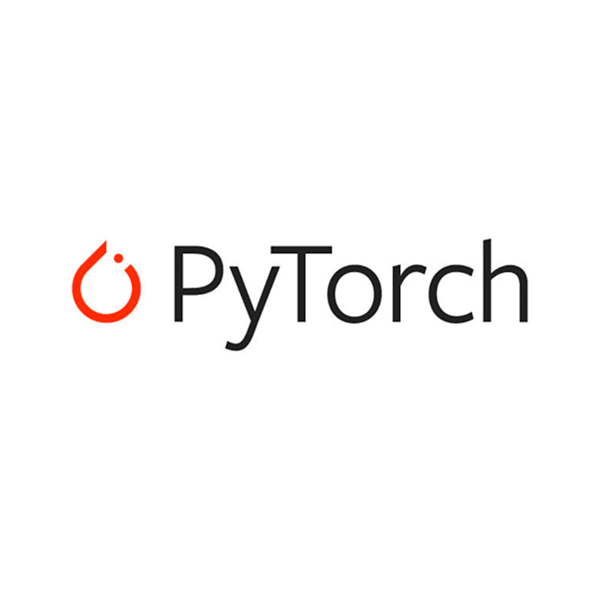
ML model clustering
ML model clustering is the process of identifying similar examples and grouping them together. You combine data from different features to create a similarity measurement. One example is a book that has three covers. As the number of features increases, the algorithm becomes more complex. The algorithm can even recognize similar items based solely on the frequency with which books are purchased. The goal of ML Model Clustering is to identify the most efficient way to group data into groups that will maximize revenues and minimize costs.
The best way to train an ML-model is to use the appropriate clustering technique. A large dataset is the best way to train the model. This will enable you to use the model to make predictions about the data that you have collected. Clustering is useful for identifying patterns and structures that exist in data that may otherwise be unrelated. It is especially useful when it comes to data science. Predictive analytics is incomplete without ML model clustering.
Unsupervised vs. supervised learning
The difference between unsupervised and supervised learning is in how they use data sets with few or none labels. While supervised learning requires the addition of labels to the data, models that are unsupervised can be trained in a way that does not require labeling. Unsupervised learning can also be used to solve problems like clustering and anomaly detection.
Although both have advantages, supervised learning algorithms work well for situations where the input and output data are known. Unsupervised learning is more flexible and can handle huge volumes of data in real time. It can also identify patterns in data which are crucial for many applications including segmentation of potential customers. A cluster of apples can be identified by an unsupervised clustering algorithm, which may identify similar features. This method can be used to tackle complex response variables like stress levels.
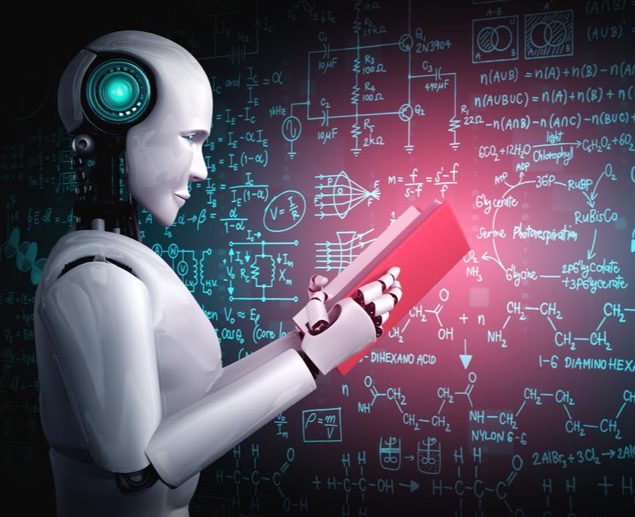
Metadata registry
The foundation of a semantic Web is a metadata registry. The idea behind this technology is to allow Web applications to share unambiguous meanings between themselves. In order to achieve this, registries will have to be multilingual, both in terms of UI and data. This requirement was incorporated into the design of metadata registry prototypes. The Dublin Core element set currently supports fourteen languages. Six languages were initially chosen to demonstrate concept development. These included languages with single byte character sets such as Spanish and double byte character sets such as Japanese. For proof of concept, however, only a small portion of each prototype could be translated.
A metadata registry is a central repository of terms used in a system. Data in a metadata register can be linked back to terms in implementers' schemas. Computer programs can also use ontologies through the metadata registry. Furthermore, registries can also be a way to reuse existing terms. Metadata registries are a great way for users to get better data.
FAQ
Are there any AI-related risks?
It is. There always will be. AI could pose a serious threat to society in general, according experts. Others argue that AI is necessary and beneficial to improve the quality life.
The biggest concern about AI is the potential for misuse. It could have dangerous consequences if AI becomes too powerful. This includes things like autonomous weapons and robot overlords.
AI could take over jobs. Many people worry that robots may replace workers. Others believe that artificial intelligence may allow workers to concentrate on other aspects of the job.
For example, some economists predict that automation may increase productivity while decreasing unemployment.
What are the benefits of AI?
Artificial Intelligence is a revolutionary technology that could forever change the way we live. It has already revolutionized industries such as finance and healthcare. It's predicted that it will have profound effects on everything, from education to government services, by 2025.
AI is being used already to solve problems in the areas of medicine, transportation, energy security, manufacturing, and transport. The possibilities for AI applications will only increase as there are more of them.
So what exactly makes it so special? It learns. Computers learn independently of humans. Instead of being taught, they just observe patterns in the world then apply them when required.
AI stands out from traditional software because it can learn quickly. Computers can read millions of pages of text every second. Computers can instantly translate languages and recognize faces.
Artificial intelligence doesn't need to be manipulated by humans, so it can do tasks much faster than human beings. It may even be better than us in certain situations.
2017 was the year of Eugene Goostman, a chatbot created by researchers. The bot fooled dozens of people into thinking it was a real person named Vladimir Putin.
This shows how AI can be persuasive. AI's adaptability is another advantage. It can be trained to perform different tasks quickly and efficiently.
Businesses don't need to spend large amounts on expensive IT infrastructure, or hire large numbers employees.
AI: Is it good or evil?
AI is seen both positively and negatively. The positive side is that AI makes it possible to complete tasks faster than ever. We no longer need to spend hours writing programs that perform tasks such as word processing and spreadsheets. Instead, instead we ask our computers how to do these tasks.
On the other side, many fear that AI could eventually replace humans. Many believe that robots may eventually surpass their creators' intelligence. This may lead to them taking over certain jobs.
Statistics
- In the first half of 2017, the company discovered and banned 300,000 terrorist-linked accounts, 95 percent of which were found by non-human, artificially intelligent machines. (builtin.com)
- That's as many of us that have been in that AI space would say, it's about 70 or 80 percent of the work. (finra.org)
- In 2019, AI adoption among large companies increased by 47% compared to 2018, according to the latest Artificial IntelligenceIndex report. (marsner.com)
- The company's AI team trained an image recognition model to 85 percent accuracy using billions of public Instagram photos tagged with hashtags. (builtin.com)
- According to the company's website, more than 800 financial firms use AlphaSense, including some Fortune 500 corporations. (builtin.com)
External Links
How To
How to setup Siri to speak when charging
Siri can do many things, but one thing she cannot do is speak back to you. This is because your iPhone does not include a microphone. If you want Siri to respond back to you, you must use another method such as Bluetooth.
Here's how Siri will speak to you when you charge your phone.
-
Under "When Using Assistive touch", select "Speak when locked"
-
To activate Siri, double press the home key twice.
-
Siri will respond.
-
Say, "Hey Siri."
-
Just say "OK."
-
Speak up and tell me something.
-
Say "I am bored," "Play some songs," "Call a friend," "Remind you about, ""Take pictures," "Set up a timer," and "Check out."
-
Say "Done."
-
Thank her by saying "Thank you"
-
Remove the battery cover (if you're using an iPhone X/XS).
-
Insert the battery.
-
Place the iPhone back together.
-
Connect the iPhone and iTunes
-
Sync the iPhone
-
Set the "Use toggle" switch to On